Enhancing Remote Sensing Image Resolution Using Convolutional Neural Networks
Abstract
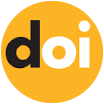
Keywords
References
Y. Sun, W. Dong, and Y. Chen, “An Improved Routing Algorithm Based on Ant Colony Optimization in Wireless Sensor Networks,” IEEE Commun. Lett., vol. 21, no. 6, pp. 1317–1320, 2017.
H. Liu, C. Li, S. He, W. Shi, Y. Chen, and W. Shi, “Simulated Annealing Particle Swarm Optimization for a Dual-input Broadband GaN Doherty like Load-Modulated Balance Amplifier Design,” IEEE Trans. Circuits Syst. II Express Briefs, vol. 69, no. 9, pp. 3734–3738, 2022.
A. Awad, A. Hawash, and B. Abdalhaq, “A Genetic Algorithm (GA) and Swarm-Based Binary Decision Diagram (BDD) Reordering Optimizer Reinforced With Recent Operators,” IEEE Trans. Evol. Comput., vol. 27, no. 3, pp. 535–549, 2023.
M. Xu, Y. Zhang, Y. Fan, Y. Chen, and D. Song, “Linear Spectral Mixing Model-Guided Artificial Bee Colony Method for Endmember Generation,” IEEE Geosci. Remote Sens. Lett., vol. 17, no. 12, pp. 2145–2149, 2020.
J. M. Mendel and D. Wu, “Critique of "a new look at type-2 fuzzy sets and type-2 fuzzy logic systems,” IEEE Trans. Fuzzy Syst., vol. 25, no. 3, pp. 725–727, 2017.
Y. Chen, Q. Mao, B. Wang, P. Duan, B. Zhang, and Z. Hong, “Privacy-Preserving Multi-Class Support Vector Machine Model on Medical Diagnosis,” IEEE J. Biomed. Heal. Informatics, vol. 26, no. 7, pp. 3342–3353, 2022.
D. B. Strydom, “Industrial Application of a Real- Time Exper t System,” The Transactions Of The Sa Institute Of Electrical Engineers. vol. 81, Issue. 2. pp. 1–6, 1990.
J. Supardi and S.-J. Horng, “Very small image face recognition using deep convolution neural networks,” J. Phys. Conf. Ser:1196 012020 ., vol. 1196, Pp. 1-6., 2019.
J. Supardi and A. S. Utami, “Development of Artificial Neural Network Architecture for Face Recognition in Real Time,” Int. J. Mach. Learn. Comput., vol. 4, no. 1, pp. 1–4, 2014.
A. Krizhevsky and G. E. Hinton, “ImageNet Classification with Deep Convolutional Neural Networks,” Advances in Neural Information Processing Systems 25 (NIPS 2012) pp. 1–9. [online available] https://proceedings.neurips.cc/paper/2012/file/c399862d3b9d6b76c8436e924a68c45b-Paper.pdf (last access: Nov. 20,2023)
M. D. Zeiler and R. Fergus, “Visualizing and Understanding Convolutional Networks,” 2014 European Conference on Computer Vision, Part I, LNCS 8689, pp. 818–833, 2014.
C. Szegedy, S. Reed, P. Sermanet, V. Vanhoucke, and A. Rabinovich, “Going deeper with convolutions,” arXiv:1409.4842 pp. 1–12. [online available] https://arxiv.org/pdf/1409.4842.pdf (last access November 20, 2022).
K. He, X. Zhang, S. Ren, and J. Sun, “Deep Residual Learning for Image Recognition,” 2015. arXiv:1512.03385 [online available] https://arxiv.org/pdf/1512.03385.pdf (last access November 20, 2022.
Vitaly Bushaev, “Adam — latest trends in deep learning optimization”, 22 October 2018. [Online]. Available: https://towardsdatascience.com/adam-latest-trends-in-deep-learning-optimization-6be9a291375c. [Access: 30 May 2023]
C. Li, D. He, X. Liu, Y. Ding, and S. Wen, “Adapting Image Super-Resolution State-of-the-arts and Learning Multi-model Ensemble for Video Super-Resolution.” 2019 IEEE conference Computer Vision Pattern Recognition (CVPR), 2019, pp. 1-8.
R.Timofte, R. Rothe, L.V. Gool, “Seven ways to improve example-based single image super resolution.” pp. 1-9, 2015, arXiv:1511.02228 [online available] https://arxiv.org/pdf/1511.02228.pdf (last access July 12, 2022).
T. G. Dietterich, “Ensemble Methods in Machine Learning,” In: Multiple Classifier Systems. MCS 2000. Lecture Notes in Computer Science, vol 1857. Springer, Berlin, Heidelberg.
L. Wang, Z. Huang, Y. Gong, and C. Pan, “Ensemble-based deep networks for image super-resolution,” Pattern Recognit., vol. 68, pp. 191–198, 2017.
J. Wu, “Introduction to Convolutional Neural Networks,” pp. 1–31, 2017, [Online vailable] https://pdfs.semanticscholar.org/450c/ a19932fcef1ca6d0442cbf52fec38fb9d1e5.pdf (last access 10 December 2019).
Z. Zhang, “Derivation of Backpropagation in Convolutional Neural Network,” pp. 1-7, 2016. [Online Available] https://pdfs.semanticscholar.org/ 5d79/11c93dd.cb34cac088d99bd0cae9124e5dcd1.pdf. (last access 26 December 2020).
Y. LeCun, L. Bottou, Y. Bengio, and P. Haffner, “Gradient-based learning applied to document recognition,” Proceedings of the IEEE, 1998., vol. 86, no. 11, pp. 2278–2324.
D. P. Kingma and J. L. Ba, “Adam: A method for stochastic optimization,” in 3rd International Conference for Learning Representations, San Diego, 2015, 2015, pp. 1–15,
S. J. Horng, J. Supardi, W. Zhou, C. T. Lin, and B. Jiang, “Recognizing Very Small Face Images Using Convolution Neural Networks,” IEEE Trans. Intell. Transp. Syst., vol. 23, no. 3, pp. 2103–2115, 2022.
J. Yang, J. Wright, T. S. Huang, and Y. Ma, “Image super-resolution via sparse representation,” IEEE Trans. Image Processing., vol. 19, no. 11, pp. 2861–2873, 2010.
M. Maire, C. Fowlkes, and J. Malik, “Contour Detection and Hierarchical Image Segmentation,” IEEE Trans. Pattern Anal. Mach. Intell., vol. 33, no. 5, pp. 898–916, 2011..
C. Dong, C. C. Loy, K. He, and X. Tang, “Image Super-Resolution Using Deep Convolutional Networks,” arXiv:1501.00092 pp. 1–14, 2014. [online available] https://arxiv.org/pdf/1501.00092.pdf (last accessed November 20, 2022.
D. Huang and H. Liu, “Face Hallucination Using Convolutional Neural Network with Iterative Back Projection,” in Proc. 11th Chinese Conference on Biometric Recognition, 2016, pp. 167–175.
Q. Cao, L. Lin, Y. Shi, X. Liang, and G. Li, “Attention-aware face hallucination via deep reinforcement learning,” Proc. - 30th IEEE Conf. Comput. Vis. Pattern Recognition, CVPR 2017, vol. 2017-Janua, 2017,.pp. 1656–1664.
J. Yamanaka, S. Kuwashima, and T. Kurita, “Fast and Accurate Image Super Resolution by Deep CNN with Skip Connection and Network in Network,” arXiv: 1707.05425, pp. 1–9. [online available] https://arxiv.org/ftp/arxiv/papers/1707/1707.05425.pdf (last accessed November 20, 2022.
Article Metrics
Metrics powered by PLOS ALM
Refbacks
- There are currently no refbacks.
Copyright (c) 2024 National Research and Innovation Agency

This work is licensed under a Creative Commons Attribution-NonCommercial-ShareAlike 4.0 International License.