Object Detection Approach Using YOLOv5 For Plant Species Identification
Abstract
In the modern era of agriculture and horticulture, biodiversity conservation requires plant species identification skills, and automatic detection is a challenging and interesting task. However, many factors often make some people mistaken in recognizing plant species that have unique and varied visual characteristics, making manual identification difficult. This problem requires an effective and accurate model for identifying plant species. So this research aims to produce a model to identify plant species that are effective and have a high level of accuracy. This research offers the use of the YOLOv5 algorithm method. The training process with epoch 200 and 53 minutes with a total of 1,220 images. Based on the results of the model performance test, the mAP value was 85.73%, precision 98.27%, and recall 94.36%. During testing, the model can identify plant species accurately on single objects and multiple objects. The results of this research show that the proposed method is successful in identifying plant species accurately.
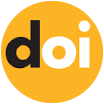
Keywords
References
K. Wang, “PANet : Few-Shot Image Semantic Segmentation with Prototype Alignment,” pp. 9197–9206.
Y. Tian, G. Yang, Z. Wang, H. Wang, E. Li, and Z. Liang, “Apple detection during different growth stages in orchards using the improved YOLO-V3 model,” Comput. Electron. Agric., vol. 157,pp.417-426,2019,doi:https://doi.org/10.1016/j.compag.2019.01.012.
I. H. Sarker, M. H. Furhad, and R. Nowrozy, “AI-Driven Cybersecurity: An Overview, Security Intelligence Modeling and Research Directions,” SN Comput. Sci., vol. 2, 2021, [Online]. Available:https://api.semanticscholar.org/CorpusID:233329532
I. Sarker, M. Hoque, K. Uddin, and T. Alsanoosy, “Mobile Data Science and Intelligent Apps: Concepts, AI-Based Modeling and Research Directions,” Mob. Networks Appl., vol. 26, 2021, doi: 10.1007/s11036-020-01650-z.
P. Akhtar et al., “Detecting fake news and disinformation using artificial intelligence and machine learning to avoid supply chain disruptions,” Ann. Oper. Res., vol. 327, no. 2, pp. 633–657, 2023, doi: 10.1007/s10479-022-05015-5.
R. Kaur, D. Gabrijelčič, and T. Klobučar, “Artificial intelligence for cybersecurity: Literature review and future research directions,” Inf. Fusion, vol. 97, p. 101804,2023,doi:https://doi.org/10.1016/j.inffus.2023.101804.
P. Jiang, D. Ergu, F. Liu, Y. Cai, and B. Ma, “A Review of Yolo Algorithm Developments,” Procedia Comput. Sci., vol. 199, pp. 1066–1073, 2022,doi:https://doi.org/10.1016/j.procs.2022.01.135.
J. Redmon and A. Farhadi, “YOLO9000: Better, Faster, Stronger,” in 2017 IEEE Conference on Computer Vision and Pattern Recognition (CVPR), 2017,pp.6517–6525.doi: 10.1109/CVPR.2017.690.
J. Redmon, S. Divvala, R. Girshick, and A. Farhadi, “You Only Look Once: Unified, Real-Time Object Detection,” in 2016 IEEE Conference on Computer Vision and Pattern Recognition (CVPR), 2016, pp. 779–788. doi: 10.1109/CVPR.2016.91.
A. M. Roy, J. Bhaduri, T. Kumar, and K. Raj, “WilDect-YOLO: An efficient and robust computer vision-based accurate object localization model for automated endangered wildlife detection,” Ecol. Inform.,vol.75,p.101919,2023,doi:https://doi.org/10.1016/j.ecoinf.2022.101919.
J. Wu and J. Dong, “applied sciences A Lightweight YOLOv5 Optimization of Coordinate Attention,” 2023.
D. Wu, S. Lv, M. Jiang, and H. Song, “Using channel pruning-based YOLO v4 deep learning algorithm for the real-time and accurate detection of apple flowers in natural environments,” Comput. Electron. Agric., vol. 178, no. July, p. 105742,2020,doi:10.1016/j.compag.2020.105742.
M. I. Mardiyah, “Implementasi Deep Learning untuk Image Classification Menggunakan Algoritma Convolutional Neural Network (CNN) Pada Citra Kebun dan Sawah,” Univ. Islam Indones.,no.June,2020,doi:10.13140/RG.2.2.10880.53768.
Sahla Muhammed Ali, “Comparative Analysis of YOLOv3, YOLOv4 and YOLOv5 for Sign Language Detection,” Int. J. Adv. Res. Innov. Ideas Educ., vol. 7, no. 4, p. 2021, 2021, [Online]. Available: www.ijariie.com2393
S. Srivastava, A. V. Divekar, C. Anilkumar, I. Naik, V. Kulkarni, and V. Pattabiraman, “Comparative analysis of deep learning image detection algorithms,” J. Big Data, vol. 8, no. 1, 2021, doi: 10.1186/s40537-021-00434-w.
M. Afonso, A. Mencarelli, G. Polder, R. Wehrens, D. Lensink, and N. Faber, “Detection of Tomato Flowers from Greenhouse Images Using Colorspace Transformations,” in Progress in Artificial Intelligence, P. Moura Oliveira, P. Novais, and L. P. Reis, Eds., Cham: Springer International Publishing, 2019, pp. 146–155.
A. Paszke, A. Chaurasia, S. Kim, and E. Culurciello, “ENet: A Deep Neural Network Architecture for Real-Time Semantic Segmentation,” Jun. 2016, Accessed: May 08, 2024.[Online].Available:http://arxiv.org/abs/1606.02147
L. Liu et al., “Deep Learning for Generic Object Detection : A Survey,” Int. J. Comput. Vis., 2019, doi: 10.1007/s11263-019-01247-4.
Y. Lecun, L. Bottou, Y. Bengio, and P. Haffner, “Gradient-based learning applied to document recognition,” Proc. IEEE, vol. 86, no. 11, pp. 2278–2324, 1998, doi: 10.1109/5.726791.
N. Sharma, V. Jain, and A. Mishra, “An Analysis Of Convolutional Neural Networks For Image Classification,” Procedia Comput. Sci., vol. 132, pp.377–384,2018,doi:https://doi.org/10.1016/j. procs.2018.05.198.
A. Karpathy, G. Toderici, S. Shetty, T. Leung, R. Sukthankar, and L. Fei-Fei, “Large-Scale Video Classification with Convolutional Neural Networks,” in 2014 IEEE Conference on Computer Vision and Pattern Recognition, 2014, pp. 1725–1732. doi: 10.1109/CVPR.2014.223.
J. Redmon, S. Divvala, R. Girshick, and A. Farhadi, “You Only Look Once : Unified , Real-Time Object Detection”.
H. Yuan, Z. Lu, R. Zhang, J. Li, S. Wang, and J. Fan, “An effective graph embedded YOLOv5 model for forest fire detection,” Comput. Intell., vol. 40, no. 2, p. e12640, Apr. 2024, doi: https://doi.org/10.1111/coin.12640.
Shengjie Cheng, P. Zhou, Yu Liu, Hongji Ma, A. Aysa, and K. Ubul, “Research on knowledge distillation algorithm based on Yolov5 attention mechanism,” Expert Syst. Appl., vol. 240, p. 122553,2024,doi:https://doi.org/10.1016/j.eswa.2023.122553.
T. Pessoa, R. Medeiros, T. Nepomuceno, G.-B. Bian, V. H. C. Albuquerque, and P. P. Filho, “Performance Analysis of Google Colaboratory as a Tool for Accelerating Deep Learning Applications,” IEEE Access, vol. PP, p. 1, Oct. 2018, doi: 10.1109/ACCESS.2018.2874767.
T. Carneiro, R. V. Medeiros Da NóBrega, T. Nepomuceno, G.-B. Bian, V. H. C. De Albuquerque, and P. P. R. Filho, “Performance Analysis of Google Colaboratory as a Tool for Accelerating Deep Learning Applications,” IEEE Access, vol. 6, pp. 61677–61685, 2018, doi: 10.1109/ACCESS.2018.2874767.
R. Xu, H. Lin, K. Lu, L. Cao, and Y. Liu, “A forest fire detection system based on ensemble learning,” Forests, vol. 12, no. 2, pp. 1–17, 2021, doi: 10.3390/f12020217.
M. Horvat, L. Jelečević, and G. Gledec, A comparative study of YOLOv5 models performance for image localization and classification. 2022.
M. H. Hamzenejadi and H. Mohseni, “Fine-tuned YOLOv5 for real-time vehicle detection in UAV imagery: Architectural improvements and performance boost,” Expert Syst. Appl., vol. 231, p.120845,2023,doi:https://doi.org/10.1016/j.eswa.2023.120845.
H. Eslamiat, “Comparing YOLOv3, YOLOv4 and YOLOv5 for Autonomous Landing Spot Detection in Faulty UAVs,” 2022.
C. Wang and H. M. Liao, “YOLOv4: Optimal Speed and Accuracy of Object Detection”.
T.-Y. Lin, P. Dollár, R. Girshick, K. He, B. Hariharan, and S. Belongie, “Feature Pyramid Networks for Object Detection,” in 2017 IEEE Conference on Computer Vision and Pattern Recognition (CVPR), 2017, pp. 936–944. doi: 10.1109/CVPR.2017.106.
W. Wang et al., “Efficient and Accurate Arbitrary-Shaped Text Detection with Pixel Aggregation Network”.
M. N. Bramasta, “Prototipe Sistem Pengenalan Pelat Kendaraan Otomatis Berbasis YOLO pada Mekanisme Pintu Masuk Departemen Elektro UNHAS,” no. May, 2022.
J. Redmon and A. Farhadi, “YOLOv3: An Incremental Improvement,” CoRR, vol. abs/1804.02767, 2018, [Online]. Available: http://arxiv.org/abs/1804.02767
C. Guo, X. Lv, Y. Zhang, and M. Zhang, “Improved YOLOv4-tiny network for real-time electronic component detection,” Sci. Rep., vol. 11, no. 1, p. 22744, 2021, doi: 10.1038/s41598-021-02225-y.
P. Ding, H. Qian, and S. Chu, “SlimYOLOv4: lightweight object detector based on YOLOv4,” J. Real-Time Image Process., vol. 19, no. 3, pp. 487–498, 2022, doi: 10.1007/s11554-022-01201-7.
Y. Song, Q.-K. Pan, L. Gao, and B. Zhang, “Improved non-maximum suppression for object detection using harmony search algorithm,” Appl. Soft Comput., vol. 81, p. 105478, 2019, doi: https://doi.org/10.1016/j.asoc.2019.05.005.
C. Guo, M. Cai, N. Ying, H. Chen, J. Zhang, and D. Zhou, “ANMS: attention-based non-maximum suppression,” Multimed. Tools Appl., vol. 81, no. 8, pp. 11205–11219, 2022, doi: 10.1007/s11042-022-12142-5.
B. B. Dursa and K. K. Tune, “Developing Traffic Congestion Detection Model Using Deep Learning Approach : A Case Study of Addis Ababa City Road,” no. September, 2023.
Article Metrics
Metrics powered by PLOS ALM
Refbacks
- There are currently no refbacks.
Copyright (c) 2024 National Research and Innovation Agency

This work is licensed under a Creative Commons Attribution-NonCommercial-ShareAlike 4.0 International License.