Ground Penetrating Radar Data Inversion Using Dual-Input Convolutional Autoencoder for Ferroconcrete Inspection
Abstract
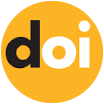
Keywords
References
H. M. Jol, Ground Penetrating Radar Theory and Applications, Elsevier. 2008.
A. Benedetto, and L. Pajewski, eds. Civil engineering applications of ground penetrating radar. Springer, 2015.
M. Nishimoto, B.P.A. Rohman, and Y. Naka. "Estimation of concrete corrosion state using ultra-wideband radar signatures." IGARSS 2019-2019 IEEE International Geoscience and Remote Sensing Symposium. IEEE, 2019.
B.P.A. Rohman, M. Nishimoto, and K. Ogata. "Material Permittivity Estimation Using Analytic Peak Ratio of Air-Coupled GPR Signatures." IEEE Access, vol. 10, pp. 13219-13228, 2022.
B. P. A. Rohman, M. Nishimoto, "Multi-scaled power spectrum based features for landmine detection using Ground Penetrating Radar," 2017 International Conference on Signals and Systems (ICSigSys), Bali, Indonesia, 2017, pp. 83-86.
S. Busch, et al. "Quantitative conductivity and permittivity estimation using full-waveform inversion of on-ground GPR data." Geophysics, vol. 77, no. 6, pp.H79-H91, 2012.
E. Forte, et al. "Velocity analysis from common offset GPR data inversion: theory and application to synthetic and real data." Geophysical Journal International, vol. 197, no. 3, pp. 1471-1483, 2014.
Z. Huang, J. Zhang. "Determination of parameters of subsurface layers using GPR spectral inversion method." IEEE Transactions on Geoscience and Remote Sensing, vol. 52, no. 12, pp. 7527-7533, 2014.
S. Jazayeri, et al. "Reinforced concrete mapping using full-waveform inversion of GPR data." Construction and Building Materials, vol. 229, pp. 117102, 2019.
M.T. Pham, S. Lefèvre. "Buried object detection from B-scan ground penetrating radar data using Faster-RCNN." IGARSS 2018-2018 IEEE International Geoscience and Remote Sensing Symposium. IEEE, 2018.
K. Dinh, N. Gucunski, T. H. Duong. "An algorithm for automatic localization and detection of rebars from GPR data of concrete bridge decks." Automation in Construction, vol. 89, pp. 292-298, 2018.
X. Xu, Y. Lei, and F. Yang. "Railway subgrade defect automatic recognition method based on improved Faster R-CNN." Scientific Programming, 2018.
Z. Tong, J. Gao, and H. Zhang. "Recognition, location, measurement, and 3D reconstruction of concealed cracks using convolutional neural networks." Construction and Building Materials, vol. 146, pp. 775-787, 2017.
R. Indrawijaya and B.P.A. Rohman, "Cognitive Linear Trajectory on GPR Imaging Using Lightweight Machine Learning," 2023 International Conference on Radar, Antenna, Microwave, Electronics, and Telecommunications (ICRAMET), Bandung, Indonesia, pp. 436-439, 2023.
J.K. Alvarez, S. Kodagoda. "Application of deep learning image-to-image transformation networks to GPR radargrams for sub-surface imaging in infrastructure monitoring." 2018 13th IEEE Conference on Industrial Electronics and Applications (ICIEA). IEEE, 2018.
B. Liu, et al. "GPRInvNet: Deep learning-based ground-penetrating radar data inversion for tunnel linings." IEEE Transactions on Geoscience and Remote Sensing, vol. 59, no. 10, pp. 8305-8325, 2021.
Y. Ji, et al. "Deep neural network-based permittivity inversions for ground penetrating radar data." IEEE Sensors Journal, vol. 21, no. 6, pp. 8172-8183, 2021
S. Yang, et al. "Defect segmentation: Mapping tunnel lining internal defects with ground penetrating radar data using a convolutional neural network." Construction and Building Materials, vol. 319, pp. 125658, 2022.
I. Goodfellow, Y. Bengio, and A. Courville. Deep Learning http://www.deeplearningbook.org, MIT Press, 2016. (accessed on 1 November 2020)
C. Warren, A. Giannopoulos, and I. Giannakis. “Gprmax: open source software to simulate electromagnetic wave propagation for ground penetrating radar,” Computer Physics Communications, vol. 209, pp. 163-170, 2016.
Article Metrics
Metrics powered by PLOS ALM
Refbacks
- There are currently no refbacks.
Copyright (c) 2024 National Research and Innovation Agency

This work is licensed under a Creative Commons Attribution-NonCommercial-ShareAlike 4.0 International License.