Deep Neural Network Classifier for Analysis of the Debrecen Diabetic Retinopathy Dataset
Abstract
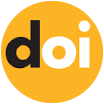
Keywords
References
T. O. Oladele, R. O. Ogundokun, A. A. Kayode, A. A. Adegun, and M. O. Adebiyi, Application of Data Mining Algorithms for Feature Selection and Prediction of Diabetic Retinopathy, vol. 11623 LNCS. Springer International Publishing, 2019. doi: 10.1007/978-3-030-24308-1_56.
I. Odeh, M. Alkasassbeh, and M. Alauthman, “Diabetic retinopathy detection using ensemble machine learning,” 2021 Int. Conf. Inf. Technol. ICIT 2021 - Proc., pp. 173–178, 2021, doi: 10.1109/ICIT52682.2021.9491645.
S. P. Dewi, N. Nurwati, and E. Rahayu, “Penerapan data mining untuk prediksi penjualan produk terlaris menggunakan metode k-nearest neighbor,” Build. Informatics, Technol. Sci., vol. 3, no. 4, pp. 639–648, 2022, doi: 10.47065/bits.v3i4.1408.
I. Romli and A. T. Zy, “Penentuan jadwal overtime dengan klasifikasi data karyawan menggunakan algoritma c4.5,” J. Sains Komput. Inform. (J-SAKTI, vol. 4, no. 2, pp. 694–702, 2020.
N. Gundluru et al., “Enhancement of detection of diabetic retinopathy using harris hawks optimization with deep learning model,” Comput. Intell. Neurosci., vol. 2022, 2022, doi: 10.1155/2022/8512469.
D. H. Firdaus, B. Imran, L. D. Bakti, and E. Suryadi, “Klasifikasi penyakit katarak berdasarkan citra menggunakan metode convolutional neural network (cnn) berbasis web,” J. Kecerdasan Buatan dan Teknol. Inf., vol. 1, no. 3, pp. 18–26, 2022.
M. Alizal, M. Susanto, A. Setyawan, H. Fitriawan, and M. Mardiana, “SISTEM keamanan ruangan dengan human detection menggunakan sensor kamera berbasis deep learning,” J. Teknoinfo, vol. 18, no. 1, pp. 182–192, 2024.
W. ANDRIAN, “KLASIFIKASI diabetic retinopathy menggunakan arsitektur deep learning model cnn (convolutional neural network).” Universitas Teknologi Digital Indonesia, 2023.
J. F. Ath-Thaariq, R. A. Rajagede, and R. Rahmadi, “Kajian pustaka pengembangan aplikasi mobile untuk pengenalan bahasa isyarat berbasis deep learning,” AUTOMATA, vol. 2, no. 1, 2021.
T. R. Gadekallu et al., “Early detection of diabetic retinopathy using pca-firefly based deep learning model,” Electron., vol. 9, no. 2, pp. 1–16, 2020, doi: 10.3390/electronics9020274.
T. R. Gadekallu, N. Khare, S. Bhattacharya, S. Singh, P. K. R. Maddikunta, and G. Srivastava, “Deep neural networks to predict diabetic retinopathy,” J. Ambient Intell. Humaniz. Comput., no. 0123456789, 2020, doi: 10.1007/s12652-020-01963-7.
D. Das, S. K. Biswas, and S. Bandyopadhyay, A critical review on diagnosis of diabetic retinopathy using machine learning and deep learning, vol. 81, no. 18. Multimedia Tools and Applications, 2022. doi: 10.1007/s11042-022-12642-4.
D. S. O. Panggabean, E. Buulolo, and N. Silalahi, “Penerapan data mining untuk memprediksi pemesanan bibit pohon dengan regresi linear berganda,” JURIKOM (Jurnal Ris. Komputer), vol. 7, no. 1, p. 56, 2020, doi: 10.30865/jurikom.v7i1.1947.
B. Antal and A. Hajdu, “Diabetic retinopathy debrecen,” UC Irvine Machine Learning Repository, 2014. https://archive.ics.uci.edu/dataset/329/diabetic+retinopathy+debrecen
J. A. ALzubi, B. Bharathikannan, S. Tanwar, R. Manikandan, A. Khanna, and C. Thaventhiran, “Boosted neural network ensemble classification for lung cancer disease diagnosis,” Appl. Soft Comput. J., vol. 80, pp. 579–591, 2019, doi: 10.1016/j.asoc.2019.04.031.
D. N. Fathurrahman, A. B. Osmond, and R. E. Saputra, “Deep neural network untuk pengenalan ucapan pada bahasa sunda dialek tengah timur ( majalengka ) deep neural network for speech recognition on sundanese language of the middle east dialect,” vol. 5, no. 3, pp. 6073–6080, 2018.
C. I. Agustyaningrum, R. Dahlia, and O. Pahlevi, “Comparison of conventional machine learning and deep neural network algorithms in the prediction of monkey-pox,” vol. 5, no. 3, 2023, [Online]. Available: https://ieeexplore.ieee.org/abstract/document/8878095
I. Elujide, S. G. Fashoto, B. Fashoto, E. Mbunge, S. O. Folorunso, and J. O. Olamijuwon, “Application of deep and machine learning techniques for multi-label classification performance on psychotic disorder diseases,” Informatics Med. Unlocked, vol. 23, p. 100545, 2021, doi: 10.1016/j.imu.2021.100545.
M. Nabipour, P. Nayyeri, H. Jabani, S. S., and A. Mosavi, “Predicting stock market trends using machine learning and deep learning algorithms via continuous and binary data; a comparative analysis,” IEEE Access, vol. 8, pp. 150199–150212, 2020, doi: 10.1109/ACCESS.2020.3015966.
A. C. Sitepu and M. Sigiro, “Analisis fungsi aktivasi relu dan sigmoid menggunakan optimizer sgd dengan representasi mse pada model backpropagation,” J. Tek. Inform. Komput. Univers., vol. 1, no. 1, pp. 12–25, 2021.
M. Astiningrum, M. Mentari, and R. R. N. Rachma, “Deteksi kesegaran daging sapi berdasarkan,” Semin. Inform. Apl., pp. 217–222, 2019.
M. Syam, I. J. Raharjo, and R. Patmasari, “Identifikasi asal daerah berdasarkan suara manusia dengan metode linier predictive coding ( lpc ) identification of regional origin based on human voice using linear predictive coding ( lpc ) method,” e-Proceeding Eng., vol. 6, no. 3, pp. 10226–10233, 2019, [Online]. Available: https://openlibrarypublications.telkomuniversity.ac.id/index.php/engineering/article/view/11346
Halprin Abhirawa, Jondri, and Anditya Arifianto, “Pengenalan wajah menggunakan convolutional neural network face,” eProceedings Eng., vol. 4, no. 3, pp. 4907–4916, 2017, [Online]. Available: https://openlibrarypublications.telkomuniversity.ac.id/index.php/engineering/article/view/5420/5400
C. D. Suhendra and A. C. Saputra, “Penentuan parameter learning rate selama pembelajaran jaringan syaraf tiruan,” vol. 14, no. 2, pp. 202–212, 2020.
Y. Wang, J. Liu, and J. Mišić, “Assessing optimizer impact on dnn model sensitivity to adversarial examples,” vol. 7, pp. 152767–152776, 2019, doi: 10.1109/ACCESS.2019.2948658.
N. A. Putro, R. Septian, W. Widiastuti, M. Maulidah, and H. F. Pardede, “Prediction of hotel booking cancellation using deep neural network and logistic regression algorithm,” J. Techno Nusa Mandiri, vol. 18, no. 1, pp. 1–8, 2021, doi: 10.33480/techno.v18i1.2056.
S. Mandt, M. D. Hof Fman, and D. M. Blei, “Stochastic gradient descent as approximate bayesian inference,” J. Mach. Learn. Res., vol. 18, pp. 1–35, 2017.
M. Wildan Putra Aldi, Jondri, and A. Aditsania, “Analisis dan implementasi long short term memory neural network untuk prediksi harga bitcoin,” e-Proceeding Eng., vol. Vol. 5, no. 2, pp. 3548–3555, 2018.
Y. Christian, “Comparison of machine learning algorithms using weka and sci-kit learn in classifying online shopper intention,” vol. 1, no. Juli, pp. 58–66, 2019, doi: 10.31289/jite.v3i1.2599.
L. Ratnawati and D. R. Sulistyaningrum, “Penerapan random forest untuk mengukur tingkat keparahan penyakit,” J. Sains Dan Seni Its, vol. 8, no. 2, pp. A71–A77, 2019.
M. A. As Sarofi, I. Irhamah, and A. Mukarromah, “Identifikasi genre musik dengan menggunakan metode random forest,” J. Sains dan Seni ITS, vol. 9, no. 1, pp. 79–86, 2020, doi: 10.12962/j23373520.v9i1.51311.
C. I. Agustyaningrum, M. Haris, R. Aryanti, and T. Misriati, “Online shopper intention analysis using conventional machine learning and deep neural network classification algorithm,” J. Penelit. Pos dan Inform., vol. 11, no. 1, pp. 89–100, 2021, doi: 10.17933/jppi.v11i1.341.
S. I. Gultom, “Implementasi data mining menentukan pola hidup sehat bagi pengguna kb menggunakan algoritma adaboost ( studi kasus : dinas serdang bedagai ),” J. Inf. dan Teknol. Ilm., vol. 7, no. 3, pp. 298–304, 2020.
Article Metrics
Metrics powered by PLOS ALM
Refbacks
- There are currently no refbacks.
Copyright (c) 2024 National Research and Innovation Agency

This work is licensed under a Creative Commons Attribution-NonCommercial-ShareAlike 4.0 International License.