Comparison of YOLOv3-tiny and YOLOv4-tiny in the Implementation Handgun, Shotgun, and Rifle Detection Using Raspberry Pi 4B
Abstract
Criminal activities frequently involve carryable weapons such as handguns, shotguns, and rifle classes. Frequently, the targets of these weapons that are captured are concealed from plain sight by the people of the crowd. The detection process for these weapons can be assisted by using deep learning. In this case, we intend to identify the model of the firearm that was detected. This research aims to apply one of the deep learning concepts, namely You Only Look Once (YOLO). The authors use versions of YOLOv3-tiny and Yolov4-tiny for the detection and classification of types of weapons, which are one of the fastest and most accurate methods of object detection, outperforming other detection algorithms. However, both require heavy computer architecture. Therefore, YOLOv3-tiny and YOLOv4-tiny, lighter versions of YOLOv3, can be solutions for smaller architectures. YOLOv3-tiny and YOLOv4-tiny have higher FPS, which is supposed to yield faster performance. Since YOLOv3-tiny and YOLOv4-tiny are modified versions of YOLOv3, the accuracy is improved, and YOLOv3 is already outperforming Faster Single Shot Detector (SSD) and Faster Region with Convolutional Neural Network (R-CNN). The authors employ YOLOv3-tiny and YOLOv4-tiny due to the fact that the Frame Per Second (FPS) and Mean Average Precision (mAP) performance of both approaches are superior in object detection. The study found that YOLOv3-tiny had a high FPS and low mAP performance: an average Intersection over Union (IoU) score of 71.54%, an accuracy of 90%, a recall score of 78%, an F1 score of 84%, and an mAP of 86.7%. While YOLOv4-tiny has low FPS and high mAP: an average IoU score of 73.19%, an accuracy of 90%, a recall score of 84%, an F1 score of 87%, and an mAP of 90.7%.
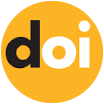
Keywords
References
J. Celentano and E. Abdelfattah, “Analyzing Gun Violence in the United States,” in 2020 11th IEEE Annual Ubiquitous Computing, Electronics & Mobile Communication Conference (UEMCON), 2020, pp. 258–261. doi: 10.1109/UEMCON51285.2020.9298154.
“2023 World Population Review. Gun Violance,” Amnesty International, 2023. [Online]. Available: https://www.amnesty.org/en/what-we-do/arms-control/gun-violence/
D. Hemenway and M. Miller, “Gun Threats Against and Self-defense Gun Use by California Adolescents,” Arch. Pediatr. Adolesc. Med., vol. 158, no. 4, pp. 395–400, Apr. 2004, doi: 10.1001/archpedi.158.4.395.
Z. Q. Zhao, P. Zheng, S. T. Xu, and X. Wu, “Object Detection with Deep Learning: A Review,” IEEE Trans. Neural Networks Learn. Syst., vol. 30, no. 11, pp. 3212–3232, 2019, doi: 10.1109/TNNLS.2018.2876865.
W. Fang, L. Wang, and P. Ren, “Tinier-YOLO: A Real-Time Object Detection Method for Constrained Environments,” IEEE Access, vol. 8, pp. 1935–1944, 2020, doi: 10.1109/ACCESS.2019.2961959.
J. Redmon, S. Divvala, R. Girshick, and A. Farhadi, “You only look once: Unified, real-time object detection,” Proc. IEEE Comput. Soc. Conf. Comput. Vis. Pattern Recognit., vol. 2016–Decem, pp. 779–788, 2016, doi: 10.1109/CVPR.2016.91.
J. Redmon and A. Farhadi, “YOLO9000: Better, faster, stronger,” Proc. - 30th IEEE Conf. Comput. Vis. Pattern Recognition, CVPR 2017, vol. 2017–Janua, pp. 6517–6525, 2017, doi: 10.1109/CVPR.2017.690.
J. Redmon and A. Farhadi, “YOLOv3: An Incremental Improvement,” 2018, [Online]. Available: http://arxiv.org/abs/1804.02767
A. Bochkovskiy, C.-Y. Wang, and H.-Y. M. Liao, “YOLOv4: Optimal Speed and Accuracy of Object Detection,” 2020, [Online]. Available: http://arxiv.org/abs/2004.10934
A. Warsi, M. Abdullah, M. N. Husen, M. Yahya, S. Khan, and N. Jawaid, “Gun Detection System Using Yolov3,” in 2019 IEEE International Conference on Smart Instrumentation, Measurement and Application (ICSIMA), 2019, pp. 1–4. doi: 10.1109/ICSIMA47653.2019.9057329.
R. M. Alaqil, J. A. Alsuhaibani, B. A. Alhumaidi, R. A. Alnasser, R. D. Alotaibi, and H. Benhidour, “Automatic Gun Detection From Images Using Faster R-CNN,” in 2020 First International Conference of Smart Systems and Emerging Technologies (SMARTTECH), 2020, pp. 149–154. doi: 10.1109/SMART-TECH49988.2020.00045.
Y. Deng, R. Campbell, and P. Kumar, “Fire and Gun Detection Based on Sematic Embeddings,” in 2022 IEEE International Conference on Multimedia and Expo Workshops (ICMEW), 2022, pp. 1–4. doi: 10.1109/ICMEW56448.2022.9859303.
M. Dextre, O. Rosas, J. Lazo, and J. C. Gutiérrez, “Gun Detection in Real-Time, using YOLOv5 on Jetson AGX Xavier,” in 2021 XLVII Latin American Computing Conference (CLEI), 2021, pp. 1–7. doi: 10.1109/CLEI53233.2021.9640100.
Y. A. Zenebe, L. Xiaoyu, W. Chao, W. Yi, H. A. Endris, and M. N. Fanose, “Towards Automatic 2D Materials Detection Using YOLOv7,” in 2022 19th International Computer Conference on Wavelet Active Media Technology and Information Processing (ICCWAMTIP), 2022, pp. 1–5. doi: 10.1109/ICCWAMTIP56608.2022.10016569.
H. Zhao, H. Zhang, and Y. Zhao, “YOLOv7-sea: Object Detection of Maritime UAV Images based on Improved YOLOv7,” in 2023 IEEE/CVF Winter Conference on Applications of Computer Vision Workshops (WACVW), 2023, pp. 233–238. doi: 10.1109/WACVW58289.2023.00029.
A. Saxena, “An Introduction to Convolutional Neural Networks,” Int. J. Res. Appl. Sci. Eng. Technol., vol. 10, no. 12, pp. 943–947, 2022, doi: 10.22214/ijraset.2022.47789.
I. Khurram, M. M. Fraz, M. Shahzad, and N. M. Rajpoot, “Dense-CaptionNet: a Sentence Generation Architecture for Fine-grained Description of Image Semantics,” Cognit. Comput., vol. 13, no. 3, pp. 595–611, 2021, doi: 10.1007/s12559-019-09697-1.
M. Everingham, L. Van Gool, C. K. I. Williams, J. Winn, and A. Zisserman, “The Pascal Visual Object Classes (VOC) Challenge,” Int. J. Comput. Vis., vol. 88, no. 2, pp. 303–338, 2010, doi: 10.1007/s11263-009-0275-4.
C. L. Zitnick and P. Dollár, “Edge Boxes: Locating Object Proposals from Edges BT - Computer Vision – ECCV 2014,” 2014, pp. 391–405.
Article Metrics
Metrics powered by PLOS ALM
Refbacks
- There are currently no refbacks.
Copyright (c) 2024 National Research and Innovation Agency

This work is licensed under a Creative Commons Attribution-NonCommercial-ShareAlike 4.0 International License.