The Effect of Window Size and Window Shape in STFT for Pre-Processing FMCW Radar Data in Human Activity Recognition Based on Bi-LSTM
Abstract
Many studies use radars for Human Activity Recognition (HAR), and numerous techniques for preprocessing FMCW radar data have been explored to improve HAR performances. Our approach employs 1-D radar to classify four human activities, i.e., walking, standing, crouching, and sitting. We use Fast Fourier Transform (FFT) and Short-Time Fourier Transform (STFT) with Kaiser window to generate range-time and Doppler-time data from inphase and quadrature radar signal. The choice of windowing parameters, i.e., window size and window shape represented by the beta parameter in Kaiser window, is considered to have significant impacts on the performances of deep learning LSTM models, including the F1-score. However, our study in this paper, including statistical analysis using t-tests, shows otherwise. Our results consistently support the null hypothesis, which mean that variations in window size and window shape do not significantly affect the F1-score. In essence, our findings underscore the robustness of our preprocessing methodology, emphasizing the stability and reliability of the selected configurations. This research provides valuable insights into the preprocessing techniques for radar data in the context of human activity recognition, enhancing the consistency and credibility of deep learning models in this domain.
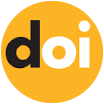
Keywords
References
. S.-W. Kang et al., “Identification of Human Motion Using Radar Sensor in an Indoor Environment,” Sensors, vol. 21, no. 7, p. 2305, Mar. 2021, doi: 10.3390/S21072305.
. S.Z. Gurbuz, S. Z. and M.G. Amin, M. G., “Radar-based human-motion recognition with deep learning: Promising applications for indoor monitoring,” IEEE Signal Processing Magazine, vol. 36, no. 4, pp. 16-28, Jul. 2019
. Y. Ren et al., “Deformable faster r-cnn with aggregating multi-layer features for partially occluded object detection in optical remote sensing images,” Remote Sensing, vol. 10, no. 9, p. 1470, Sept. 2018, doi: 10.3390/rs10091470.
. A. Markman et al., “Three-dimensional object visualization and detection in low light illumination using integral imaging,” Optics letters, vol. 42, no. 16, pp. 3068-3071, Aug. 2017.
. W. Bouachir et al., “Intelligent video surveillance for real-time detection of suicide attempts,” Pattern Recognition Letters, vol. 110, pp. 1-7, Jul. 2018.
. R.R. Subramanian and V. Vasudevan, “HARDeep: design and evaluation of a deep ensemble model for human activity recognition,” International Journal of Innovative Computing and Applications, vol. 14, no. 3, pp. 155–166, 2023, doi: 10.1504/IJICA.2023.131356.
. M.H. Arshad et al, “Human Activity Recognition: Review, Taxonomy and Open Challenges,” Sensors, vol. 22, no. 17, p. 6463, Aug. 2022, doi: 0.3390/s22176463.
. M. G. Amin, Ed., Radar for indoor monitoring: Detection, classification, and assessment. Boca Raton, FL, USA: CRC Press Taylor & Francis Group, 2017.
. C Karabacak et al., “Knowledge exploitation for human micro-Doppler classification,” IEEE Geoscience and Remote Sensing Letters, vol. 12, no. 10, pp. 2125-2129, Oct. 2015.
. Y. Kim and T. Moon, “Human detection and activity classification based on micro-Doppler signatures using deep convolutional neural networks,” IEEE geoscience and remote sensing letters, vol. 13, no. 1, pp. 8-12, Jan. 2016.
. C. Ding, et al., “Continuous human motion recognition with a dynamic range-Doppler trajectory method based on FMCW radar,” IEEE Transactions on Geoscience and Remote Sensing, vol. 57, no. 9, pp. 6821-6831, Sep. 2019.
. X. Qiao, et al., “Human activity classification based on moving orientation determining using multistatic micro-Doppler radar signals,” IEEE Transactions on Geoscience and Remote Sensing, vol. 60, pp. 1-15, 2022.
. C. Li, et al., “A review on recent advances in Doppler radar sensors for noncontact healthcare monitoring,” IEEE Transactions on microwave theory and techniques, vol. 61, no. 5, pp. 2046-2060, May 2013
. W. Ding, X. Guo, and G. Wang, “Radar-Based Human Activity Recognition Using Hybrid Neural Network Model with Multidomain Fusion,” IEEE Trans Aerosp Electron Syst, vol. 57, no. 5, pp. 2889–2898, Oct. 2021
. X. Li, et al., “A survey of deep learning-based human activity recognition in radar,” Remote Sensing, vol. 11, no. 9. MDPI AG, May 2019. doi: 10.3390/rs11091068.
. S. Ahmed, J. Park, and S. H. Cho, “FMCW Radar Sensor Based Human Activity Recognition using Deep Learning,” in 2022 International Conference on Electronics, Information, and Communication (ICEIC), Feb. 2022. doi: 10.1109/ICEIC54506.2022.9748776.
. L. Cohen, “Time-frequency distributions-a review,” Proceedings of the IEEE, vol. 77, no. 7, pp. 941-981, 1989.
. R. Rafli, F. Y. Suratman, and Istiqomah, “FMCW Radar Signal Processing for Human Activity Recognition with Convolutional Neural Network,” In Proceeding of the 3rd International Conference on Electronics, Biomedical Engineering, and Health Informatics (ICEBEHI), Oct. 2022, pp. 429-445, doi: 10.1007/978-981-99-0248-4_29
. D. R. Sardjono, F. Y.Suratman, and Istiqomah, “Human Motion Change Detection Based on FMCW Radar,” 7th IEEE Asia Pacific Conference on Wireless and Mobile (APWiMob), Dec. 2022, doi: 10.1109/APWiMob56856.2022.
. M. A. Richards, Fundamentals of radar signal processing. New York: McGraw-Hill Education, 2022.
. A.V. Oppenheim, Discrete-time signal processing. Pearson Education India, 1999.
. uRAD User Manual Raspberry Pi version Software SDK v1.1., Anteral, Navvara, Spain, 2021.
. Python 3.11.0. (2022). Python.org. Accessed: Sep. 05, 2023. [Online]. Available: https://www.python.org/
. S.Z. Gurbuz, Ed., Deep Neural Network Design for Radar Applications. SciTech Publishing, 2020.
. Numpy 1.25.0. (2023). Numpy.org. Accessed: Sep. 05, 2023. [Online]. Available: https://numpy.org/
. Raspberry Pi 4 Model B Datasheet, Raspberry Pi (Trading) Ltd., United Kingdom (UK) [Online]. Available: https://datasheets.raspberrypi.com/rpi4/raspberry-pi-4-datasheet.pdf
. A. Geron, Hands-On Machine Learning with Scikit-Learn, Keras & TensorFlow. Sebastopol, CA, USA: O’Reilly, 2019.
. A. Shrestha, et al., “Continuous Human Activity Classification from FMCW Radar with Bi-LSTM Networks,” IEEE Sens J, vol. 20, no. 22, pp. 13607–13619, Nov. 2020.
Article Metrics
Metrics powered by PLOS ALM
Refbacks
- There are currently no refbacks.
Copyright (c) 2024 National Research and Innovation Agency

This work is licensed under a Creative Commons Attribution-NonCommercial-ShareAlike 4.0 International License.