Designing Human-Robot Communication in the Indonesian Language Using the Deep Bidirectional Long Short-Term Memory Algorithm
Abstract
Humanoid robots closely resemble humans and engage in various human-like activities while responding to queries from their users, facilitating two-way communication between humans and robots. This bidirectional interaction is enabled through the integration of speech-to-text and text-to-speech systems within the robot. However, research on two-way communication systems for humanoid robots utilizing speech-to-text and text-to-speech technologies has predominantly focused on the English language. This study aims to develop a real-time two-way communication system between humans and a robot, with data collected from ten respondents, including eight males and two females. The sentences used adhere to the standard rules of the Indonesian language. The speech-to-text system employs a deep bidirectional long short-term memory algorithm, coupled with feature extraction via the Mel frequency cepstral coefficients, to convert spoken language into text. Conversely, the text-to-speech system utilizes the Python pyttsx3 module to translate text into spoken responses delivered by the robot. The results indicate that the speech-to-text model achieves a high level of accuracy under quiet-room conditions, with noise levels ranging from 57.5 to 60 dB, boasting an average word error rate (WER) of 24.99% and 25.31% for speakers within and outside the dataset, respectively. In settings with engine noise and crowds, where noise levels range from 62.4 to 86 dB, the measured WER is 36.36% and 36.96% for speakers within and outside the dataset, respectively. This study demonstrates the feasibility of implementing a two-way communication system between humans and a robot, enabling the robot to respond to various vocal inputs effectively.
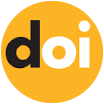
References
M. Malik, M. K. Malik, K. Mehmood, and I. Makhdoom, “Automatic speech recognition: a survey,” Multimed. Tools Appl., vol. 80, no. 6, pp. 9411–9457, 2021, doi: 10.1007/s11042-020-10073-7.
K. R. Chowdhary, Fundamentals of artificial intelligence. Springer India, 2020. doi: 10.1007/978-81-322-3972-7.
D. Khurana, A. Koli, K. Khatter, and S. Singh, “Natural language processing: state of the art, current trends and challenges,” Multimed. Tools Appl., vol. 82, no. 3, pp. 3713–3744, 2023, doi: 10.1007/s11042-022-13428-4.
A. A. Alnuaim et al., “Human-computer interaction for recognizing speech emotions using multilayer perceptron classifier,” J. Healthc. Eng., vol. 2022, 2022, doi: 10.1155/2022/6005446.
F. Del Duchetto, P. Baxter, and M. Hanheide, “Lindsey the tour guide robot - usage patterns in a museum long-term deployment,” 2019 28th IEEE Int. Conf. Robot Hum. Interact. Commun. RO-MAN 2019, 2019, doi: 10.1109/RO-MAN46459.2019.8956329.
E. J. Hwang, B. A. MacDonald, and H. S. Ahn, “End-to-end dialogue system with multi languages for hospital receptionist robot,” 2019 16th Int. Conf. Ubiquitous Robot. UR 2019, pp. 278–283, 2019, doi: 10.1109/URAI.2019.8768694.
S. L. Burewar, “Voice controlled robotic system by using fft,” 2018 4th Int. Conf. Converg. Technol. I2CT 2018, pp. 2018–2021, 2018, doi: 10.1109/I2CT42659.2018.9058098.
S. Patil, A. Abhigna, Arpitha, Deepthi, and Priyanka, “Voice controlled robot using labview,” Proc. - 2018 Int. Conf. Des. Innov. 3Cs Comput. Commun. Control. ICDI3C 2018, pp. 80–83, 2018, doi: 10.1109/ICDI3C.2018.00025.
V. Andreas, A. A. S. Gunawan, and W. Budiharto, “Anita: intelligent humanoid robot with self-learning capability using indonesian language,” 2019 4th Asia-Pacific Conf. Intell. Robot Syst. ACIRS 2019, pp. 144–147, 2019, doi: 10.1109/ACIRS.2019.8935964.
M. C. Bingol and O. Aydogmus, “Performing predefined tasks using the human–robot interaction on speech recognition for an industrial robot,” Eng. Appl. Artif. Intell., vol. 95, no. January, p. 103903, 2020, doi: 10.1016/j.engappai.2020.103903.
C. Deuerlein, M. Langer, J. Seßner, P. Heß, and J. Franke, “Human-robot-interaction using cloud-based speech recognition systems,” Procedia CIRP, vol. 97, no. March, pp. 130–135, 2020, doi: 10.1016/j.procir.2020.05.214.
M. H. Tambunan, Martin, H. Fakhruroja, Riyanto, and C. Machbub, “Indonesian speech recognition grammar using kinect 2.0 for controlling humanoid robot,” 2018 Int. Conf. Signals Syst. ICSigSys 2018 - Proc., no. 978, pp. 59–63, 2018, doi: 10.1109/ICSIGSYS.2018.8373568.
R. Rendyansyah, A. P. P. Prasetyo, and S. Sembiring, “Voice command recognition for movement control of a 4-dof robot arm,” Elkha, vol. 14, no. 2, p. 118, 2022, doi: 10.26418/elkha.v14i2.57556.
Z. He, “Improving lstm based acoustic model with dropout method,” Proc. - 2019 Int. Conf. Artif. Intell. Adv. Manuf. AIAM 2019, pp. 27–30, 2019, doi: 10.1109/AIAM48774.2019.00012.
W. Ying, L. Zhang, and H. Deng, “Sichuan dialect speech recognition with deep lstm network,” Front. Comput. Sci., vol. 14, no. 2, pp. 378–387, 2020, doi: 10.1007/s11704-018-8030-z.
R. Shashidhar, S. Patilkulkarni, and S. B. Puneeth, “Combining audio and visual speech recognition using lstm and deep convolutional neural network,” Int. J. Inf. Technol., vol. 14, no. 7, pp. 3425–3436, 2022, doi: 10.1007/s41870-022-00907-y.
J. Yi, H. Ni, Z. Wen, B. Liu, and J. Tao, “CTC regularized model adaptation for improving lstm rnn based multi-accent mandarin speech recognition,” Proc. 2016 10th Int. Symp. Chinese Spok. Lang. Process. ISCSLP 2016, no. October 2016, 2017, doi: 10.1109/ISCSLP.2016.7918420.
R. W. Pratiwi, Y. Sari, and Y. Suyanto, “Attention-based bilstm for negation handling in sentimen analysis,” IJCCS (Indonesian J. Comput. Cybern. Syst., vol. 14, no. 4, p. 397, 2020, doi: 10.22146/ijccs.60733.
M. Ravanelli, T. Parcollet, and Y. Bengio, “The Pytorch-Kaldi speech recognition toolkit,” ICASSP, IEEE Int. Conf. Acoust. Speech Signal Process. - Proc., vol. 2019-May, pp. 6465–6469, 2019, doi: 10.1109/ICASSP.2019.8683713.
S. Dwijayanti, M. A. Tami, and B. Y. Suprapto, “Speech-to-text conversion in indonesian language using a deep bidirectional long short-term memory algorithm,” Int. J. Adv. Comput. Sci. Appl., vol. 12, no. 3, pp. 225–230, 2021, doi: 10.14569/IJACSA.2021.0120327.
A. Graves, N. Jaitly, and A. Mohamed, “Hybrid speech recognition with deep bidirectional LSTM," in 2013 IEEE workshop on automatic speech recognition and understanding, pp. 273–278, 2013.
K. Kurniawan, “Indonesian nlp resources,” 2018. [Online]. Available: https://github.com/kmkurn/id-nlp-resource. (Oct 15, 2021)
Y. Y. Wang, A. Acero, and C. Chelba, “Is word error rate a good indicator for spoken language understanding accuracy,” 2003 IEEE Work. Autom. Speech Recognit. Understanding, ASRU 2003, pp. 577–582, 2003, doi: 10.1109/ASRU.2003.1318504.
J. Grosman, “HuggingSound: a toolkit for speech-related tasks based on hugging face’s tools,” 2022. [Online]. Availble: https://github.com/jonatasgrosman/huggingsound (December 15, 2023).
L. Cristoforetti, M. Ravanelli, M. Omologo, A. Sosi, A. Abad, M. Hagmuller, and P. Maragos, “The DIRHA simulated corpus,” Proc. 9th Int. Conf. Lang. Resour. Eval. Lr. 2014, pp. 2629–2634, 2014.
J. Barker, R. Marxer, E. Vincent, and S. Watanabe, “The third ‘CHiME’ speech separation and recognition challenge : dataset, task, and baselines," In 2015 IEEE Workshop on Automatic Speech Recognition and Understanding (ASRU), pp. 504–511, 2015.
V. Panayotov, G. Chen, D. Povey, and S. Khudanpur, “Librispeech: an asr corpus based on public domain audio books,” ICASSP, IEEE Int. Conf. Acoust. Speech Signal Process. - Proc., vol. 2015-August, pp. 5206–5210, 2015, doi: 10.1109/ICASSP.2015.7178964.
Debbie Clason, “Understanding the degrees of hearing loss.” [Online]. Available: https://www.healthyhearing.com/report/41775-Degrees-of-hearing-loss (April 7, 2023)
Article Metrics
Metrics powered by PLOS ALM
Refbacks
- There are currently no refbacks.
Copyright (c) 2024 National Research and Innovation Agency

This work is licensed under a Creative Commons Attribution-NonCommercial-ShareAlike 4.0 International License.