Two-Stage Object Detection for Autonomous Vehicles With VGG-16 Based Faster R-CNN
Abstract
The implementation of object detection for autonomous vehicles is essential as it is necessary to identify common object on the street so proper response could be designed. While single stage object may be smaller in computations, two-stage object detection is preferred due to the ability to localize the object. In this paper, we propose to use Faster R-CNN with VGG-16 backbone for detections of object on the street. We evaluate the method with open image subset by selecting objects that are common on street. We explore several hyper-parameters setup such as learning rate and the number of ROI regions to find the optimum set-up. We found that the use of learning rate 10-6 with Adam optimizer to be the optimum value for this task. We also found that increasing the number of ROI may benefit the performance. This shows that there is potential for getting a higher mAP with increase the amount of RoI.
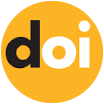
Keywords
References
C. Peng, K. Zhao, and B. C. Lovell, “Faster ILOD: Incremental learning for object detectors based on faster RCNN,” Pattern Recognit. Lett., vol. 140, 2020, doi: 10.1016/j.patrec.2020.09.030.
A. Boukerche and Z. Hou, “Object detection using deep learning methods in traffic scenarios,” ACM Comput. Surv., vol. 54, no. 2, pp. 1–35, 2021.
M. Carranza-García, J. Torres-Mateo, P. Lara-Benítez, and J. García-Gutiérrez, “On the performance of one-stage and two-stage object detectors in autonomous vehicles using camera data,” Remote Sens., vol. 13, no. 1, 2021, doi: 10.3390/rs13010089.
Q. Fan, L. Brown, and J. Smith, “A closer look at Faster R-CNN for vehicle detection,” in IEEE Intelligent Vehicles Symposium, Proceedings, 2016. doi: 10.1109/IVS.2016.7535375.
T. A. Shaikh, W. A. Mir, T. Rasool, and S. Sofi, “Machine Learning for Smart Agriculture and Precision Farming: Towards Making the Fields Talk,” Archives of Computational Methods in Engineering, vol. 29, no. 7. 2022. doi: 10.1007/s11831-022-09761-4.
G. Litjens et al., “A survey on deep learning in medical image analysis,” Medical Image Analysis, vol. 42. 2017. doi: 10.1016/j.media.2017.07.005.
A. Rehman, M. Ahmed Butt, and M. Zaman, “A Survey of Medical Image Analysis Using Deep Learning Approaches,” in Proceedings - 5th International Conference on Computing Methodologies and Communication, ICCMC 2021, 2021. doi: 10.1109/ICCMC51019.2021.9418385.
P. Soviany and R. T. Ionescu, “Optimizing the trade-off between single-stage and two-stage deep object detectors using image difficulty prediction,” in Proceedings - 2018 20th International Symposium on Symbolic and Numeric Algorithms for Scientific Computing, SYNASC 2018, 2018. doi: 10.1109/SYNASC.2018.00041.
R. Girshick, J. Donahue, T. Darrell, and J. Malik, “Rich feature hierarchies for accurate object detection and semantic segmentation,” Proc. IEEE Comput. Soc. Conf. Comput. Vis. Pattern Recognit., pp. 580–587, 2014, doi: 10.1109/CVPR.2014.81.
S. S. A. Zaidi, M. S. Ansari, A. Aslam, N. Kanwal, M. Asghar, and B. Lee, “A survey of modern deep learning based object detection models,” Digit. Signal Process. A Rev. J., vol. 126, pp. 1–18, 2022, doi: 10.1016/j.dsp.2022.103514.
Z. Zou, K. Chen, Z. Shi, Y. Guo, and J. Ye, “Object Detection in 20 Years: A Survey,” Proc. IEEE, pp. 1–39, 2023, doi: 10.1109/JPROC.2023.3238524.
R. Girshick, “Fast R-CNN,” Proc. IEEE Int. Conf. Comput. Vis., vol. 2015 Inter, pp. 1440–1448, 2015, doi: 10.1109/ICCV.2015.169.
S. Ren, K. He, R. Girshick, and J. Sun, “Faster R-CNN: Towards Real-Time Object Detection with Region Proposal Networks,” IEEE Trans. Pattern Anal. Mach. Intell., vol. 39, no. 6, pp. 1137–1149, 2017, doi: 10.1109/TPAMI.2016.2577031.
Q. Zhang, X. Chang, Z. Meng, and Y. Li, “Equipment detection and recognition in electric power room based on faster R-CNN,” Procedia Comput. Sci., vol. 183, pp. 324–330, 2021, doi: 10.1016/j.procs.2021.02.066.
X. Liyun, L. Boyu, M. Hong, and L. Xingzhong, “Improved faster R-CNN algorithm for defect detection in powertrain assembly line,” Procedia CIRP, vol. 93, pp. 479–484, 2020, doi: 10.1016/j.procir.2020.04.031.
Z. Song, L. Fu, J. Wu, Z. Liu, R. Li, and Y. Cui, “Kiwifruit detection in field images using Faster R-CNN with VGG16,” IFAC-PapersOnLine, vol. 52, no. 30, pp. 76–81, 2019, doi: 10.1016/j.ifacol.2019.12.500.
F. Gao et al., “Multi-class fruit-on-plant detection for apple in SNAP system using Faster R-CNN,” Comput. Electron. Agric., vol. 176, no. May, p. 105634, 2020, doi: 10.1016/j.compag.2020.105634.
M. F. Rahman and B. Bambang, “Deteksi Sampah pada Real-time Video Menggunakan Metode Faster R-CNN,” Appl. Technol. Comput. Sci. J., vol. 3, no. 2, pp. 117–125, 2021, doi: 10.33086/atcsj.v3i2.1846.
R. M. Alaqil, J. A. Alsuhaibani, B. A. Alhumaidi, R. A. Alnasser, R. D. Alotaibi, and H. Benhidour, “Automatic Gun Detection from Images Using Faster R-CNN,” Proc. - 2020 1st Int. Conf. Smart Syst. Emerg. Technol. SMART-TECH 2020, pp. 149–154, 2020, doi: 10.1109/SMART-TECH49988.2020.00045.
M.-C. Roh and J. Lee, “Refining faster-RCNN for accurate object detection,” in 2017 Fifteenth IAPR International Conference on Machine Vision Applications (MVA), 2017, pp. 514–517. doi: 10.23919/MVA.2017.7986913.
M. Lokanath, K. S. Kumar, and E. S. Keerthi, “Accurate object classification and detection by faster-RCNN,” in IOP Conference Series: Materials Science and Engineering, 2017. doi: 10.1088/1757-899X/263/5/052028.
Article Metrics
Metrics powered by PLOS ALM
Refbacks
- There are currently no refbacks.
Copyright (c) 2024 National Research and Innovation Agency

This work is licensed under a Creative Commons Attribution-NonCommercial-ShareAlike 4.0 International License.