Prediction Of Myers-Briggs Type Indicator Personality Using Long Short-Term Memory
Abstract
Personality is defined as the mix of features and qualities that make up an individual's particular character, including thoughts, feelings, and behaviors. With the rapid development of technology, personality computing is becoming a popular research field by providing users with personalization. Many researchers have used social media data to automatically predict personality. This research uses a public dataset from Kaggle, namely the Myers-Briggs Personality Type Dataset. The purpose of this study is to predict the accuracy and F1-score values so that the performance for predicting and classifying Myers–Briggs Type Indicator (MBTI) personality can work optimally by using attributes from the MBTI dataset, namely posts and types. Predictive accuracy analysis was carried out using the Long Short-Term Memory (LSTM) algorithm with random oversampling technique with the Imblearn library for MBTI personality type prediction and comparing the performance of the method proposed in this study with other popular machine learning algorithms. Experiments show that the LSTM model using the RMSprop optimizer and learning speed of 10-3 provides higher performance in terms of accuracy while for the F1-score the LSTM model using the RMSprop Optimizer and learning speed of 10-2 gives a higher value than the proposed machine learning algorithm so that the model MBTI dataset using LSTM with random oversampling can help in identifying the MBTI personality type.
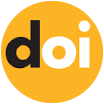
Keywords
References
M. J, (MBTI) Myers-Briggs Personality Type Dataset, 2017, Kaggle, Mar 2021. [Online]. Available: https://www.kaggle.com/datasnaek/mbti-type.
M. H. Amirhosseini and H. Kazemian, “Machine Learning Approach to Personality Type Prediction Based on the Myers–Briggs Type Indicator®,” Multimodal Technol. Interact., vol. 4, no. 1, pp. 1-15, 2020.
N. H. Z. Abidin, M. A. Remli, N. M. Ali, D. N. E. Phon, N. Yusoff, H. K. Adli and A. H. Busalim, “Improving Intelligent Personality Prediction using Muyers-Briggs Type Indocator and Random Forest Classifier,” International Journal of Advanced Computer Science and Applications, vol. 11, no. 11, pp. 192-199, 2020.
T. A. Tutupoly and I. Alfarobi, “Komparasi algoritma C4.5 dan naive bayes yang dikembangkan menjadi web intellegence pada perhitungan bonus tahunan karyawan di PT. ABC,” Jurnal Mitra Pendidikan (JMP Online), vol. 3, no. 1, pp. 92-103, 2019.
Y. Mehta, S. Fatehi, A. Kazameini, C. Stachl, E. Cambria and S. Eetemadi, “Bottom-up and top-down: predicting personality with psycholinguistic and language model features,” in 2020 IEEE International Conference on Data Mining (ICDM), Sorrento, 2020. Crossref
S. Bharadwaj, S. Sridhar, R. Choudhary and R. Srinath, “Persona traits identification based on Myers-Briggs Type Indicator (MBTI) - a text classification approach,” in 2018 International Conference on Advances in Computing, Communications and Informatics (ICACCI), Bangalore, 2018. Crossref
C. Li. et al., “Feature extraction from social media posts for psychometric typing of participants,” in Lecture Notes in Computer Science, vol. 10915, D. D. Schmorrow dan C. M. Fidopiastis (eds) Augmented Cognition: Intelligent Technologies. AC 2018, New York, Springer, Cham, 2018, pp. 267-286.
S. S. Keh and I.-T. Cheng, “Myers-briggs personality classification and personality-specific language generation using pre-trained language models,” 15 July 2019. [Online]. Available: https://arxiv.org/abs/1907.06333.
E. J. Choong and K. D. Varathan, “Predicting judging-perceiving of myers-briggs type indicator (mbti) in online social forum,” PeerJ 9:e11382, pp. 1-27, 2021.
S. Patel, M. Nimje, A. Shetty and S. Kulkarni, “Personality analysis using social media,” International Journal of Engineering Research & Technology (IJERT), vol. 09, no. 03, pp. 306-309, 2020.
M. Frković, N. Čerkez, B. Vrdoljak and S. Skansi, “Evaluation of Structural Hyperparameters for Text Classification with LSTM Networks,” in 2020 43rd International Convention on Information, Communication and Electronic Technology (MIPRO), Opatija, 2020.
R. Hernandez and I. S. Knight, “Predicting Myres-Briggs Type Indicator with text classification,” Curran Associates Inc., New York, 2017.
B. Cui and C. Qi, “Survey analysis of machine learning methods for natural language processing for MBTI personality type prediction,” 2017.
Article Metrics
Metrics powered by PLOS ALM
Refbacks
- There are currently no refbacks.
Copyright (c) 2021 National Research and Innovation Agency

This work is licensed under a Creative Commons Attribution-NonCommercial-ShareAlike 4.0 International License.