Infinite Latent Feature Selection Technique for Hyperspectral Image Classification
Abstract
The classification process is one of the most crucial processes in hyperspectral imaging. One of the limitations in classification process using machine learning technique is its complexities, where hyperspectral image format has a thousand band that can be used as a feature for learning purpose. This paper presents a comparison between two feature selection technique based on probability approach that not only can tackle the problem, but also improve accuracy. Infinite Latent Feature Selection (ILFS) and Relief Techniques are implemented in a hyperspectral image to select the most important feature or band before applied in Support Vector Machine (SVM). The result showed ILFS technique can improve classification accuracy better than Relief (92.21% vs. 88.10%). However, Relief can extract less feature to reach its best accuracy with only 6 features compared with ILFS with 9.
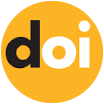
Keywords
References
X. Briottet et al., “Military applications of hyperspectral imagery,” vol. 6239, p. 62390B, 2006. Crossref
L. M. Dale et al., “Hyperspectral imaging applications in agriculture and agro-food product quality and safety control: A review,” Appl. Spectrosc. Rev., vol. 48, no. 2, pp. 142–159, 2013. Crossref
G. Lu and B. Fei, “Medical hyperspectral imaging: a review,” J. Biomed. Opt., vol. 19, no. 1, p. 010901, 2014. Crossref
J. Transon, R. d’Andrimont, A. Maugnard, and P. Defourny, “Survey of hyperspectral Earth Observation applications from space in the Sentinel-2 context,” Remote Sens., vol. 10, no. 2, pp. 1–32, 2018. Crossref
S. B. Serpico and G. Moser, “Extraction of spectral channels from hyperspectral images for classification purposes,” IEEE Trans. Geosci. Remote Sens., vol. 45, no. 2, pp. 484–495, 2007. Crossref
J. F. R. Rochac and N. Zhang, “Feature Extraction in Hyperspectral Imaging Using Adaptive Feature Selection Approach,” pp. 36–40, 2016. Crossref
S. B. Serpico and L. Bruzzone, “A new search algorithm for feature selection in hyperspectral remote sensing images,” IEEE Trans. Geosci. Remote Sens., vol. 39, no. 7, pp. 1360–1367, 2001. Crossref
M. Pal and G. M. Foody, “Feature selection for classification of hyperspectral data by SVM,” IEEE Trans. Geosci. Remote Sens., vol. 48, no. 5, pp. 2297–2307, 2010. Crossref
M. Fauvel, C. Dechesne, A. Zullo, and F. Ferraty, “Fast forward feature selection for the nonlinear classification of hyperspectral images,” Jan. 2015, [online] Available: https://arxiv.org/abs/1501.00857.
N. El Aboudi and L. Benhlima, “Review on wrapper feature selection approaches,” in Proc. of 2016 Int. Conf. Eng. MIS, (ICEMIS 2016), 2016. Crossref
N. Sánchez-Maroño, A Alonso-Betanzos, M Tombilla-Sanromán, “Filter methods for feature selection - A comparative study,” in Proc. of the Eighth International Conference on Intelligent Data Engineering and Automated Learning (IDEAL 2007), 2007, pp. 178-187. Crossref
S. Lei, “A feature selection method based on information gain and genetic algorithm,” in Proc. of 2012 International Conference on Computer Science and Electronics Engineering, 2012, vol. 2, pp. 355-358. Crossref
P. Meesad, P. Boonrawd, and V. Nuipian, “A chi-square-test for word importance differentiation in text classification,” in Proc. of International Conference on Information and Electronics Engineering, 2011, pp. 110–114.
R.J. Urbanowicz, M. Meeker, R.S. Olsona, L.C. William, and H.M. Jason, “Relief-based feature selection: introduction and review,” J. Biomed. Inform., vol. 85, pp. 189-203, September 2018. Crossref
G. Roffo, S. Melzi, U. Castellani, and A. Vinciarelli, “Infinite latent feature selection : a probabilistic latent graph-based ranking approach,” in Proc. of International Conference on Computer Vision, 2017, pp. 1398-1406. Crossref
T. Hofmann, “Probabilistic Latent Semantic Analysis,” in Proc.of the Fifteenth Conference on Uncertainty in Artificial Intelligence, 1999, pp. 289-296.
F. Melgani and L. Bruzzone, “Classification of hyperspectral remote sensing images with support vector machines,” IEEE Trans. Geosci. Remote Sens., vol. 42, no. 8, Aug. 2004. Crossref
C. Chang and C. Lin, “LIBSVM : A Library for Support Vector Machines,” ACM Trans. Intell. Syst. Technol., 2013.
L. Wenzhi, “Feature Extraction and Classification for Hyperspectral Remote Sensing Images,” Doctoral dissertation, Universiteit Gent, 2012.
G. Roffo, “Ranking to Learn and Learning to Rank: On the Role of Ranking in Pattern Recognition Applications,” June 2017, [online] Available: https://arxiv.org/pdf/1706.05933.
Hyperspectral Remote Sensing Scenes http://www.ehu.eus/ccwintco/index.php/Hyperspectral_Remote_Sensing_Scenes
Article Metrics
Metrics powered by PLOS ALM
Refbacks
- There are currently no refbacks.
Copyright (c) 2019 National Research and Innovation Agency

This work is licensed under a Creative Commons Attribution-NonCommercial-ShareAlike 4.0 International License.