Determination of the Optimum Wavelet Basis Function for Indonesian Vowel Voice Recognition
Abstract
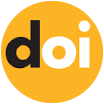
Keywords
References
M. A. Anusuya and S. K. Katti, “Front end analysis of speech recognition: a review,” Int. J. Speech Technol., vol. 14, no. 2, pp.99–145, Jan. 2011. Crossref
C. R. Rashmi, “Review of algorithms and applications in speech recognition system,” Int. J. Comput. Sci. Inf. Technol., vol. 5, no.4, pp. 5258–5262, 2014.
N. M. Mahesh S. Chavan, “Studies on implementation of Harr and Daubechies Wavelet for denoising of Speech Signal,” Int. J. Circuits, Syst. Signal Process., vol. 4, no. 3, pp. 83–96, 2010.
N. Ahuja, S. Lertrattanapanich, and N. K. Bose, “Properties determining choice of mother wavelet,” in IEE Proc.-Vis. Image Signal Process., 2005, vol. 152, no. 5. Crossref
O. Farooq and S. Datta, “Phoneme recognition using wavelet based features,” in Information Sciences, 2003, vol. 150, no. 1–2, pp. 5–15. Crossref
S. Hidayat, R. Hidayat, and T. B. Adji, “Speech recognition of KV-patterned Indonesian syllable using MFCC, wavelet and HMM,” J. Ilm. Kursor, vol. 8, no. 2, pp. 67–78, 2015. Crossref
R. Hidayat, Priyatmadi, and W. Ikawijaya, “Wavelet based feature extraction for the vowel sound,” in Proc. of The 2015 International Conference on Information Technology Systems and Innovation (ICITSI), 2015, pp. 1–4. Crossref
R. R. Coifman and M. V. Wickerhauser, “Entropy-based algorithms for best basis selection,” IEEE Trans. Inf. Theory, vol. 38, no. 2, pp. 713–718, 1992. Crossref
J. Galka and M. Ziolko, “Mean best basis algorithm for Wavelet speech parameterization,” in Proc. of Fifth International Conference on Intelligent Information Hiding and Multimedia Signal Processing, 2009, pp. 1110–1113. Crossref
J. Rafiee, M. A. Rafiee, N. Prause, and M. P. Schoen, “Wavelet basis functions in biomedical signal processing,” Expert Syst. Appl., vol. 38, no. 5, pp. 6190–6201, 2011. Crossref
J. Rafiee and P. W. Tse, “Use of autocorrelation of wavelet coefficients for fault diagnosis,” Mech. Syst. Signal Process.,vol. 23, pp. 1554–1572, 2009. Crossref
Suyanto and S. Hartati, “Design of Indonesian LVCSR Using Combined Phoneme and Syllable Models,” in The 7th International Conference on Information & Communication Technology and Systems (ICTS), 2013, pp. 191–196.
International Phonetic Association, “IPA Learning Tool.” [Online]. Available: http://www.internationalphoneticalphabet.org/ipa-learningtool/IPA-Interface/ipa-chart-with-sounds2.html. [Accessed: 01-Jan-2015].
S. Hidayat, U. Hasanah, and A. A. Rizal, “Algoritma Penghapus Derau / Silence Dan Penentuan Endpoint Dengan Nilai Ambang Terbobot Untuk Sinyal Suara,” in Seminar Nasional APTIKOM (SEMNASTIKOM), 2016, no. October 2016, pp. 320–323.
Abriyono and A. Harjoko, “Pengenalan Ucapan Suku Kata Bahasa Lisan Menggunakan Ciri LPC, MFCC, dan JST,” IJCCS, vol. 6, no. 2, pp. 23–34, 2012.
R. Asliyan, “Syllable Based Speech Recognition,” in Speech Technology, I. Ipsic, Ed. InTech, 2011, pp. 263–284. Crossref
S. Poornima, “Basic Characteristics of Speech Signal Analysis,” Int. J. Innov. Res. Dev., vol. 5, no. 4, pp. 1–5, 2016.
V. K. Ingle and J. G. Proakis, Digital Signal Processing using MATLAB, 3rd ed. USA: CENGAGE Learning, 2012.
X. Huang, A. Acero, and H.-W. Hon, Spoken Language Processing: A Guide to Theory, Algorithm and System Development. Prentice Hall, 2001.
I. McLoughlin, Applied Speech,and Audio Processing : With Matlab Examples, 1st ed. United Kingdom: Cambridge University Press, 2009.
O. Rioul and M. Vetterli, “Wavelets and Signal Processing,” IEEE SP Magazine, Oct-1991.
M. Misiti, Y. Misiti, G. Oppenheim, and J. Poggi, Wavelet Toolbox TM 4 User ’ s Guide. 2009.
I. Daubechies, “Orthonormal bases of compactly supported wavelets,” Commun. Pure Appl. Math., vol. 41, no. 7, pp. 909–996, 1988. Crossref
Article Metrics
Metrics powered by PLOS ALM
Refbacks
- There are currently no refbacks.
Copyright (c) 2017 National Research and Innovation Agency

This work is licensed under a Creative Commons Attribution-NonCommercial-ShareAlike 4.0 International License.