Classification of Radar Environment Using Ensemble Neural Network with Variation of Hidden Neuron Number
Abstract
Target detection is a mandatory task of radar system so that the radar system performance is mainly determined by its detection rate. Constant False Alarm Rate (CFAR) is a detection algorithm commonly used in radar systems. This method is divided into several approaches which have different performance in the different environments. Therefore, this paper proposes an ensemble neural network based classifier with a variation of hidden neuron number for classifying the radar environments. The result of this research will support the improvement of the performance of the target detection on the radar systems by developing such an adaptive CFAR. Multi-layer perceptron network (MLPN) with a single hidden layer is employed as the structure of base classifiers. The first step of this research is the evaluation of the hidden neuron number giving the highest accuracy of classification and the simplicity of computation. According to the result of this step, the three best structures are selected to build an ensemble classifier. On the ensemble structure, all of those three MLPN outputs then be collected and voted for getting the majority result in order to decide the final classification. The three possible radar environments investigated are homogeneous, multiple-targets and clutter boundary. According to the simulation results, the ensemble MLPN provides a higher detection rate than the conventional single MLPNs. Moreover, in the multiple-target and clutter boundary environments, the proposed method is able to show its highest performance.
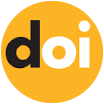
Keywords
References
M. A. Richards, Fundamentals of Radar Signal Processing. Tata McGraw-Hill Education, 2005.
J. J. Jen, “A study of CFAR implementation cost and performance tradeoffs in heterogeneous environments,” Msc.thesis, Faculty of California State Polytechnic University,Pomona, 2011.
H. Rohling, “Radar CFAR thresholding in clutter and multiple target situations,” Aerospace and Electronic Systems, IEEE Transactions , Vol. AES-19, No. 4, pp 608-621, July 1983. Crossref
P. P. Gandhi, and S. Kassam, “Analysis of CFAR processors in homogeneous background,” Aerospace and Electronic Systems, IEEE Transactions, Vol. 24, No. 4, pp 427– 445, July 1988. Crossref
B. M. Keel, “Chapter 16 Constant false alarm rate detectors,” in Principles of Modern Radar: Basic Principles. SciTech Pub.,2010.
M. E. Smith, and P. K. Varshney, “VI-CFAR: A novel CFAR algorithm based on data variability,” in Proc. IEEE National Radar Conference, pp. 263 – 268, 1997. Crossref
N.B. Gálvez, et al., “Improved neural network based CFAR detection for non homogeneous background and multiple target situations,” Latin American applied research, vol 42, no. 4, pp. 343-350, 2012.
B. P. A. Rohman, D. Kurniawan and M.T. Miftahusudur, “Switching CA/OS CFAR for radar target detection in nonhomogeneous environment,” in Proc. of 17th IEEE International Electronic Symposium, 2015. Crossref
L. Rokach, “Ensemble-based classifiers,” Artificial Intelligence Review, vol. 33, no. 1-2, pp. 1-39, 2012. Crossref
L. K. Hansen and P. Salamon, “Neural Network Ensembles,” IEEE Transactions on Pattern Analysis and Machine Intelligence, vol. 12, pp. 993-1001, Oct 1990. Crossref
A. Rahman, S. Tasnim, “Ensemble classifiers and their applications: a review,” International Journal of Computer Trends and Technology (IJCTT), Vol. 10 No.1, pp. 31-35, April 2014.
U. Naftaly, N. Intrator and D. Horn, “Optimal ensemble averaging of neural networks,” Network: Computation in Neural Systems, pp. 283-296, 1997. Crossref
I. Maqsood, M. R. Khan, A. Abraham, “An ensemble of neural networks for weather forecasting,” Neural Computing and Applications, Vol. 13, No. 2, pp 112–122, Jun. 2004. Crossref
Y. Li, S. Gao, and S. Chen, “Ensemble feature weighting based on local learning and diversity,” in Proc. 26th AAAI Conf. Artificial Intelligence, 2012, pp. 1019–1025.
P. McLeod, B. Verma, "Variable hidden neuron ensemble for mass classification in digital mammograms," IEEE Computational Intelligence Magazine, Vol. 8, Issue. 1, pp 68-76, Feb. 2013. Crossref
D. K. Barrow, S. F. Crone, "Crogging (cross-validation aggregation) for forecasting - A novel algorithm of neural network ensembles on time series subsamples," in Proc. The 2013 International Joint Conference on Neural Networks (IJCNN), 2013. Crossref
M. Pulido, P. Melin, “Ensemble neural network optimization using the particle swarm algorithm with type-1 and type-2 fuzzy integration for time series prediction,” Recent Advances on Hybrid Approaches for Designing Intelligent Systems, Springer International Publishing, 2014, pp 99-112. Crossref
M. Sekine, Y. Mao, “Weibull Radar Clutter”, United Kingdom: Peter Peregrinus Ltd., 1990.
K. Du and M. N. S. Swamy, Neural Networks And Statistical Learning, Springer Science and Business Media, 2013.
Article Metrics
Metrics powered by PLOS ALM
Refbacks
- There are currently no refbacks.
Copyright (c) 2017 National Research and Innovation Agency

This work is licensed under a Creative Commons Attribution-NonCommercial-ShareAlike 4.0 International License.